Predictive model to estimate medical school dropouts at Universidad Nacional de La Matanza
DOI:
https://doi.org/10.54789/rs.v2i1.20Keywords:
student dropouts, artificial intelligence, predictive value of testsAbstract
Introduction: The application of an Artificial Intelligence (AI) model can determine the set of socioeconomic-financial data obtained at the entrance of each student to the medicine program at Universidad Nacional de La Matanza and its relationship with voluntary dropouts.
Material and methods: A longitudinal and analytical study was carried out. Observation and analysis tools were: 1. Socioeconomic-family data from a voluntary survey of all medical students upon admission to the program in the period 2012-2018. 2. Data on the academic performance of the students during the program. An AI program called Orange Canvas was applied on the contents of the abovementioned databases, which is a data mining tool that provides a series of complements for data analysis, visualization and modelling.
Results: The "Random Forest" model of the AI program achieved a 72% accuracy, which increased to 76.7% with the Test and Score model. Finally, with the KNN model, the accuracy to predict dropouts rose to 87,2%.
Conclusion: After performing all the tests on the data set of the initial survey and the dataset of the subjects each student passed, the KNN model of the AI tool reached the highest prediction level (87,2%) for dropouts.
References
Fishbein M, Ajzen I. Attitudes toward objects as predictors of simple and multiple behavioural criteria. Psycological Review. 1975. 81:59-74.
Durkheim E. Suicide: A study in sociology (Internet) New York: Free Press. Disponible en https://www.gacbe.ac.in/images/E%20books/Durkheim%20-%20Suicide%20-%20A%20study%20in%20sociology.pdf
Cabrera A, Nora A, Castañeda M. The role of finances in the persistence process: A structural model. Res High Educ. 1992. 33:(5):303-336.
Cabrera A, Nora A, Castañeda M. College Persistence: Structural Equations Modelling Test of Integrated Model of Student Retention. J Higher Educ. 1993. 64(2):123-320.
Bernal E, Cabrera A, Terenzini P. The relationship between race and socioeconomic status (SES): Implications for institutional research and admissions policies. Removing Vestiges: Research-based strategies to promote inclusion. American Association of Community Colleges. 2000. (3):6-19.
St. John E, Cabrera A, Aker N, Asker E. Economic influences on persistence. In: J. M. Braxton. Reworking the student departure puzzle: new theory and research on college student retention. Nashville: Vanderbilt University Press. 2000. 29-47
Berger J, Milem J. Organizational Behavior in Higher Education and Student Outcomes. In: J. Smart (Ed.), Higher Education: Handbook of theory and research. 2000. (15):268-338.
Tinto V. Limits of theory and practice of student attrition. J Higher Educ. 1982. 3(6):687-700.
Spady WG. Dropouts from higher education: An interdisciplinary review and synthesis. Interchange. 1970. (1):64–85.
Nye J. Independence and Interdependence. Foreign Policy. Spring. 1976. (22):130-161.
Bean J. Student Attrition. Intensions and Confidence: Res High Educ. 1980. (17):291-320.
Bean JP, Metzner BS. A conceptual model non-traditional undergraduate student attrition. Rev Educ Res. 1985. 55(4):485-540.
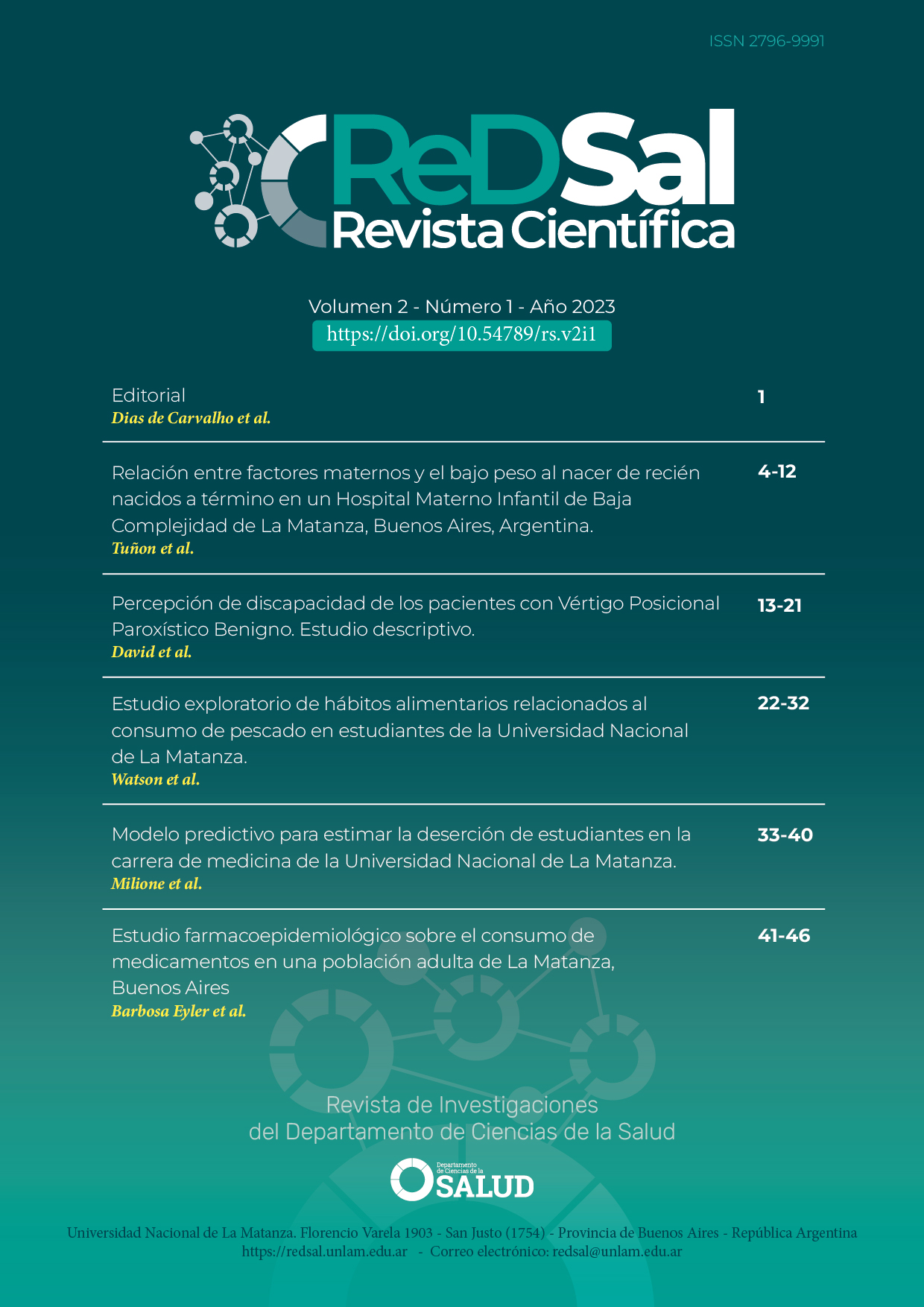
Downloads
Published
How to Cite
Issue
Section
License
Copyright (c) 2023 ReDSal

This work is licensed under a Creative Commons Attribution 4.0 International License.